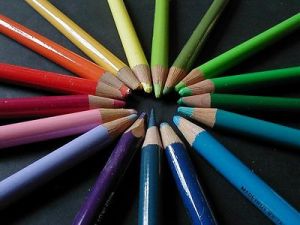
Dude, Why Is Your Name So Long?
Seems like a good punch line for my life!
If you have one of those long names that people are always struggling to pronounce and spell, welcome to my world.
I frequently collaborate with my colleagues in different geographies. Few days ago I was having similar conversation via online chatting sessions with 3 of my peers. It was interesting to see how each of these 3 folks pronounced my name differently.
Here is a quick glance –
1. Prashantha – This is a common Indian name variation where many names either end with ‘ta’ or ‘tha’ and are used alternatively.
2. Parashanta – This one is a typo because it’s a very uncommon variation of my name. I presume that this colleague was busy getting into the topic and was not really keen on getting my name right. (And, I love it when someone makes my name longer than it already is!)
On the other hand, if a non-Indian person calls me this I would have to assume they had no idea what my name spelt like. Given full consideration to what they might be going through to deal with a name like mine, I can picture them trying hard (or cursing) when they type my first name. (And even longer and complex last name. Let’s not go there).
3. Praschan – The first 4 characters of my first and last name combined together. Now this is not normal variant, but an example of what happens when people try to derive your name out of your email address or twitter handle. Similar to what some of the marketing spam emails I get which start with – “Hello Praschan, We have an irresistible (And a very stupid) offer for you”.
The last variation here did not surprise me because the person I was talking to is not an Indian and thinks ‘Praschan’ is perhaps a valid name.
Indian names are usually complex and lengthy. This is because of many reasons and is often attributed to variety of culture India has, languages people speak, religion, castes etc. India has as many as 22 official languages and even more local languages and dialects. This variety is what makes for subtle, often confusing, differences in names, naming styles and length.
Thailand is another country where I worked on MDM implementations and dealt with long names. Thai names, both first and last are often rangy because they are required to be unique. It’s rare to find two Thai people share same full name.
Similarly, during an implementation in Japan, I dealt with 2 different name formats. Customer records had both Kanji and Kana names attached to them. Specifically the Kanji variation was extremely difficult because it had characters of Chinese origin.
Names which are key ingredients of customer record cause lot of dilemma during data matching process, especially when you are dealing with international names which are long and complex.
Consider you are using deterministic matching system, when 2 records match because social security and address are same, a slight difference in name might make the system behave as if the name wasn’t a match and avoid automatic merging. However, a probabilistic engine would catch simple typos, phonetic variations and transpositions and would possibly give a higher match score, thus help resolve this case without human intervention. But we have to make a sure that the probabilistic engine we use have capabilities to handle international names and identify their variations.
The matching engines using the algorithm based on edit distances (An edit distance is the number of simple edit operations required to transform one string to another) give good results for international names. Less the edit distance, more is the similarity between two names. However the catch is, many irrelevant matches are retrieved when all name variations spell more or less the same.
Name matching on a large scale customer data with international names poses numerous challenges due to ambiguous names in multiple ethnicities. How did you deal with international names and matching!? Share your experience via comments.
COMMENTS
Leave A Comment
RECENT POSTS

Composable Applications Explained: What They Are and Why They Matter
Composable applications are customized solutions created using modular services as the building blocks. Like how...
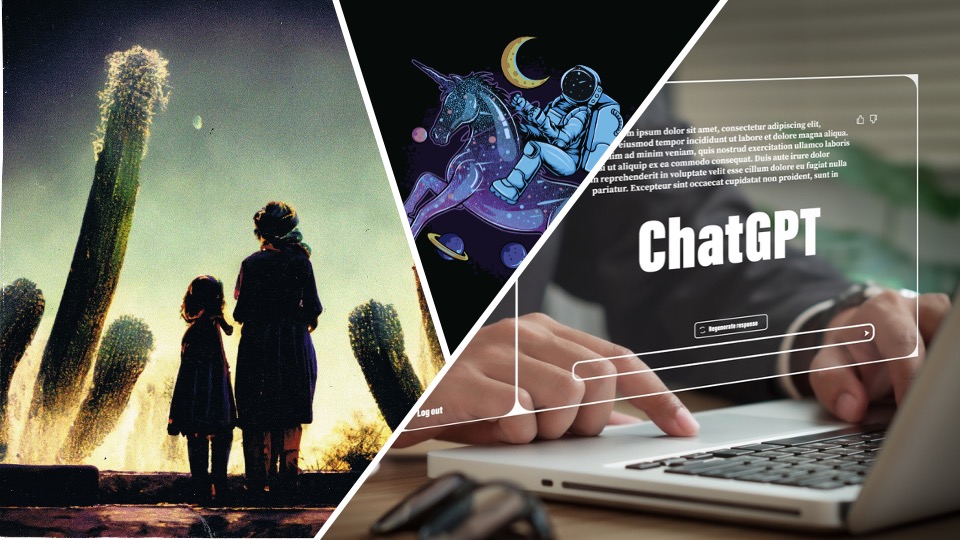
Is ChatGPT a Preview to the Future of Astounding AI Innovations?
By now, you’ve probably heard about ChatGPT. If you haven’t kept up all the latest...
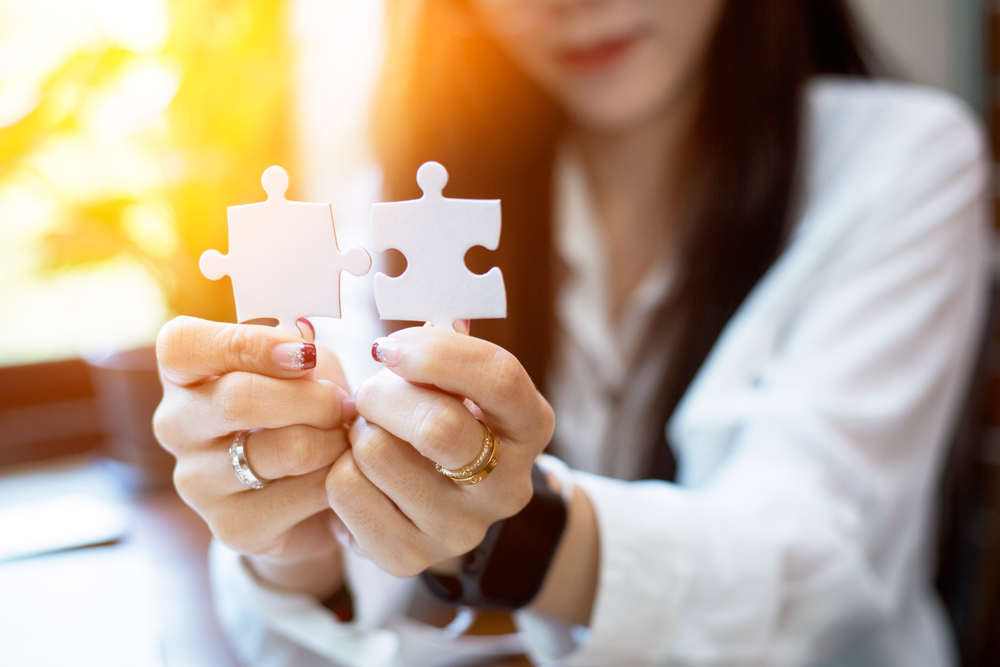
How MDM Can Help Find Jobs, Provide Better Care, and Deliver Unique Shopping Experiences
Industrial data is doubling roughly every two years. In 2021, industries created, captured, copied, and...
Slightly beside the main point of this post – I’d love to read an account of how people deal with your *last* name 😀
In general you have to combine different approaches in the same matching routine by having an algorithm (edit distance and beyond), using synonyms defined up front (nicknames, common misspellings and beyond) and probabilistic learning at the same time.
What I have seen is that many match solutions works best in the country/culture where they are born because there is an emphasize on the most frequent pain in that place, for example nicknames in the United States.
However, increasing globalization and having people like you and me living in other cultures than where we are born and given names makes the matching challenges more difficult.
When organizations are collecting names the best way is to be proactive and try to get the name right the first time wherever possible.
As Henrik correctly mentioned, there is no single algorithm that takes care of all the variations. You need to apply many matching techniques (like nicknames, abbreviations, common misspellings, transposed characters, phonetic variations etc.) but with Indian data, you are always left with a huge number of records that are not fully processed because of some new address (or name) pattern. it takes a very long time to cover most of the patterns and an efficient solution needs to have a function that takes care of these records which are only partially processed.